7 min read
Abstract
This research is committed to the quest for discovering the most effective sentiment analysis algorithms while emphasizing neutrality, non-bias, and impartiality in our evaluation. By utilizing the F1 score as a fundamental evaluation metric, our study rigorously examines the performance of diverse sentiment analysis models through a comparative analysis. Our overarching goal is to identify algorithms that excel in the impartial and unbiased discernment of sentiment in natural language text. Through this analysis, we aim to illuminate the top-performing solutions suitable for practical applications, ensuring a fair and impartial assessment.
What are the key results?
Methodology
Sampling
The reviews used to train and test the models were all generated by ChatGPT 3.5 in order to use brand new - never seen - examples, ensuring that none of the models tested (nor Lettria's) could be trained on these data.
Distribution
The 17,374 reviews generated by ChatGPT are equally balanced between 3 sentiments: Positive (POS), Neutral (NEU) and Negative (NEG) according to the following distribution:

Results assessment
In this research, we employ the F1 score as a pivotal metric for assessing the performance of our sentiment analysis model, comparing it with existing models. The F1 score, which harmonizes precision and recall, is an essential tool in evaluating the model's accuracy and its ability to correctly classify sentiments in natural language text, making it a reliable benchmark for comparison.
The F1 score in NLP deep learning quantifies model performance by balancing precision and recall, crucial for tasks like sentiment analysis or text classification. It harmonizes these metrics into a single value, aiding in model evaluation.
Detailed results
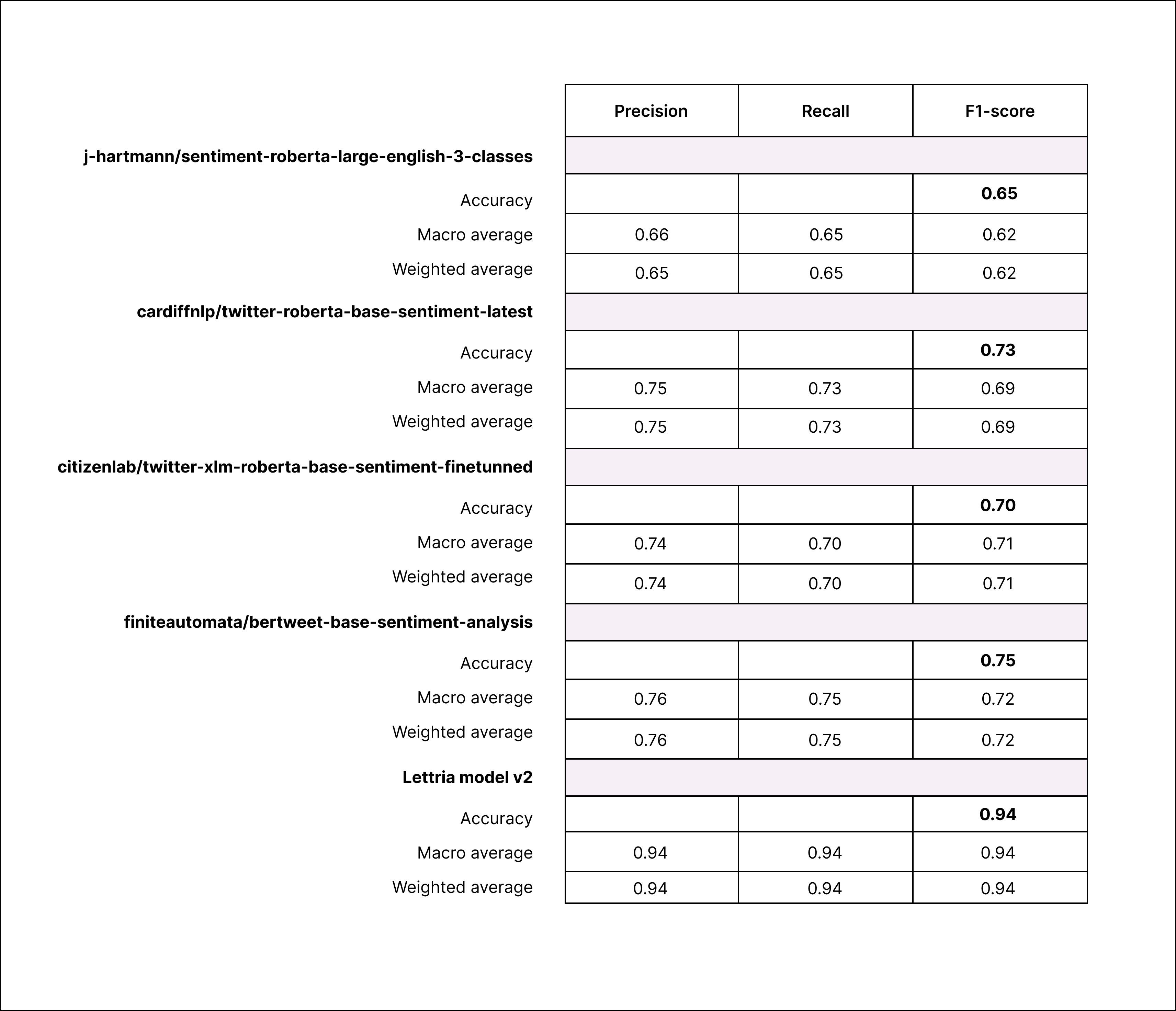
Caveats
Benchmarking sentiment analysis models over a generated sample of reviews comes with certain caveats. Firstly, generated text may not accurately represent real-world sentiments, potentially leading to biased or unrealistic evaluations. Secondly, model overfitting can occur if the generated data closely resembles the training data. Additionally, synthetic data may lack the nuances and context present in authentic reviews, affecting the model's generalization. Therefore, while it serves as a useful initial benchmark, it should be complemented with evaluations on real-world data to ensure robust model performance.
Open Data
The dataset used for our analysis is publicly accessible and can be downloaded through the following link: Download the sample.
This open dataset not only ensured the reproducibility of our research but also encouraged wider participation and scrutiny within the research community.
Open data initiatives like this one serve as catalysts for scientific progress, enabling researchers to build upon each other's work, ultimately advancing the field of sentiment analysis and natural language processing as a whole.