6 min
As businesses navigate an increasingly data-driven world, the need for smarter, faster, and more efficient data processing is paramount. Two transformative technologies have emerged as game-changers in this field: Large Language Models (LLMs) and Knowledge Graphs. When you compare LLM vs Knowledge Graph, it becomes clear that both approaches offer unique benefits that, when combined, can revolutionize how your business handles data, insights, and customer interactions. For a deeper dive into how LLMs have evolved, check out The Progress of Large Language Models: Revolutionizing NLP by Evan Strait.
In this article, we’ll explore the key differences between LLMs and Knowledge Graphs, their individual benefits, and why your business should be leveraging both.
What Is an LLM (Large Language Model)?
Large Language Models (LLMs) are advanced AI systems trained on vast datasets of text to understand and generate human-like language. These models, such as OpenAI’s GPT-4 and Google’s BERT, are designed to interpret, predict, and produce natural language—making them invaluable for tasks that require deep context and linguistic comprehension. To learn more about the evolution and capabilities of these models, visit Our Take on Large Language Models for Text Analysis.
Key Features of LLMs
- Natural Language Understanding: LLMs excel at processing human language, including nuances, context, and sentiment.
- Content Generation: They can automatically generate coherent, contextually relevant text, making them ideal for automating communication.
- Scalability: LLMs can be scaled to handle tasks across various industries—from customer service to content creation and data analysis.
Use Cases for LLMs in Business
LLMs are increasingly used to streamline processes involving language, such as:
- Customer Support Automation: Handling customer queries with instant, context-aware responses.
- Document Summarization: Automatically summarizing long documents and extracting critical insights.
- Content Creation: Supporting marketing teams by generating high-quality content at scale. Explore how businesses are leveraging LLM technology in Our Take on Large Language Models for Text Analysis.
What Is a Knowledge Graph?
A Knowledge Graph is a structured data model that organizes information as entities (nodes) and their relationships (edges). This framework allows businesses to visualize and analyze complex interconnections between data points—providing clarity on how different entities relate to one another. To see practical implementation steps, check out How to build a knowledge graph in 9 simple steps.
Key Features of Knowledge Graphs
- Relational Mapping: They provide a visual representation of complex relationships by clearly showing how data points are connected.
- Structured Data Organization: Knowledge Graphs organize information into navigable structures, making querying and analysis more efficient.
- Enhanced Data Integration: They enable businesses to merge data from multiple sources, ensuring all relevant information is interconnected.
Use Cases for Knowledge Graphs in Business
Knowledge Graphs are especially useful for businesses managing large volumes of data. Common applications include:
- Recommendation Engines: Personalizing customer experiences by linking products based on customer behavior.
- Data Integration: Combining various internal and external data sources into a unified framework.
- Advanced Search Capabilities: Delivering more precise search results by leveraging relationships between data points. For a real-world example, see Leroy Merlin: Using a Knowledge Graph to Improve Product Recommendations.
LLM vs Knowledge Graph: Key Differences
When comparing LLM vs Knowledge Graph, it’s evident that while both technologies are powerful, they serve distinct roles in data processing.
LLMs: Language-Centric Processing
LLMs are primarily designed to process and generate human-like text. Their strengths include:
- Unstructured Data Processing: Ideal for handling unstructured text—such as emails, reviews, and reports.
- Contextual Understanding: They interpret the meaning behind words to generate relevant responses.
- Adaptability: LLMs can be trained across various domains, making them versatile for numerous business applications.
Knowledge Graphs: Relationship-Centric Processing
Conversely, Knowledge Graphs focus on structuring data and exposing relationships. They excel in:
- Structured Data Management: Optimized for organizing relational data to show connections between entities.
- Efficient Querying: Allowing for quick searches, even in complex datasets.
- Data Consistency: Maintaining consistency across multiple data sources.
Summary Comparison
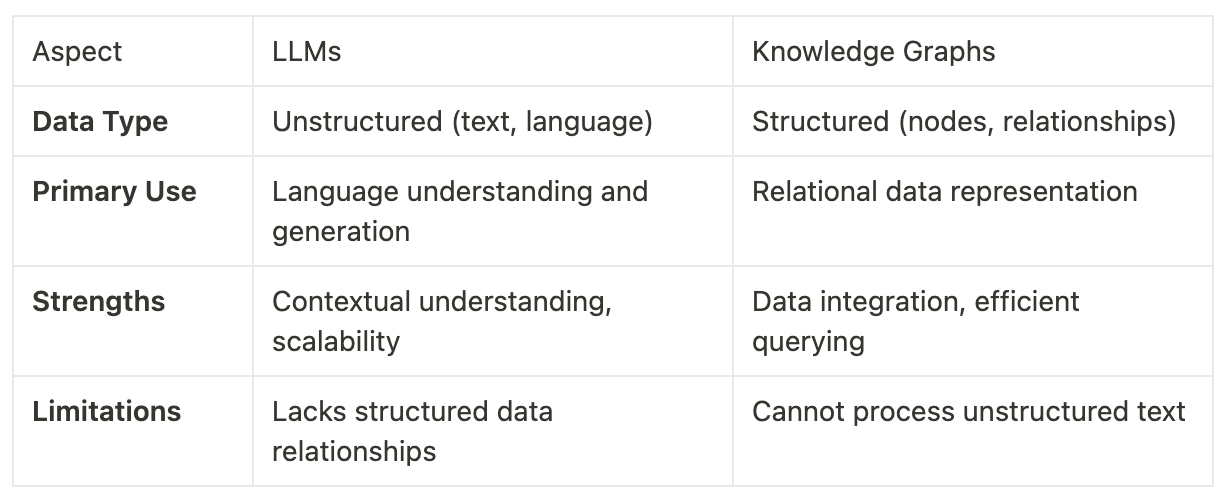
Why Your Business Needs Both LLMs and Knowledge Graphs
Although LLMs and Knowledge Graphs each serve unique purposes, their integration unlocks deeper insights and transforms data strategy.
1. Combining Unstructured and Structured Data
Businesses generate both unstructured text (like customer reviews) and structured data (such as sales records). LLMs excel at processing text, while Knowledge Graphs organize structured data into actionable insights. For example, an e-commerce platform could use an LLM to analyze customer feedback and then link that feedback to purchase history via a Knowledge Graph, providing a comprehensive view of customer behavior.
2. Enhancing Search Capabilities
LLMs can interpret complex queries and generate detailed responses, while Knowledge Graphs can filter and structure search results based on data relationships. This combination significantly improves the search experience—especially in data-heavy fields like finance. Learn more about how structured knowledge boosts search efficiency in From Chatbots to Knowledge Graphs: Why Analysts Need a New Way to Access and Analyze Data.
3. Improving Customer Interaction and Personalization
By engaging customers with conversational LLMs and reinforcing that interaction with structured data from Knowledge Graphs, businesses can deliver highly personalized experiences. For instance, a recommendation engine might use LLMs to understand customer queries while a Knowledge Graph ensures that suggestions are based on accurate purchase history and preferences.
4. Automating Complex Workflows
Integrating LLMs and Knowledge Graphs can automate workflows that require both natural language processing and relational data mapping. A legal firm, for instance, might use LLMs to summarize case files and then use a Knowledge Graph to map relationships among clients, cases, and outcomes—streamlining research and preparation.
5. Enabling More Accurate Insights and Decision-Making
LLMs provide a nuanced understanding of unstructured text, while Knowledge Graphs offer a clear map of data relationships. Together, they deliver comprehensive insights that enhance decision-making. In healthcare, LLMs could analyze patient records, and a Knowledge Graph could correlate conditions, treatments, and outcomes—leading to more personalized care plans. For more on transforming insights into action, check out 5 Best Practices to Create Powerful Ontologies with LLMs.
Conclusion
The debate of LLM vs Knowledge Graph isn’t about choosing one technology over the other; it’s about leveraging both to unlock the full potential of your data. LLMs excel at processing and generating natural language, while Knowledge Graphs provide a robust structure to map data relationships. Together, they offer a powerful solution for improving decision-making, enhancing customer interactions, and streamlining operations.
By adopting both LLMs and Knowledge Graphs, your business can stay ahead of the competition—harnessing the full power of data to drive smarter decisions and improved efficiency. To explore how this integration can transform your operations, visit our GraphRAG Blog and learn more about cutting-edge approaches in AI.