13 minute read
Introduction
In the business world, AI and machine learning is all the rage today. But did you know that in order to train machine learning and AI algorithms that show seamless and easy looking results, there is a very important step that first needs to take place? That step is data annotation.
Data annotation is the process of labeling or tagging raw data, such as text, images, or videos, to provide context and make it useful for machine learning algorithms. As artificial intelligence and machine learning applications continue to grow, the need for efficient and accurate data labeling has become increasingly important.
But there is a problem: traditionally, data annotation has been a technically intensive process. This meant that the barrier to entry for a data annotator was quite high. This was alright in the past, when the usage of AI and ML was not as high as it is today. But if AI and machine learning approaches are going to proliferate as much as they are today, we need more data annotators.
This can happen by training more people to be more technically savvy in order to use annotation tools. But that’s expensive, time consuming and not scaleable. Is there another solution?
Enter no-code data annotation platforms. These innovative solutions simplify the data annotation process by eliminating the need for programming skills, making the task more accessible to a wider range of users. As a result, these platforms are playing a crucial role in the development of AI and machine learning models.
Text annotation, a specific type of data annotation, deals with labeling and categorizing text data to enable natural language processing and understanding. In this article, we will explore the transformative impact of no-code data annotation platforms on the text annotation landscape and how they are revolutionizing the industry.
Traditional data annotation methods
Manual data labeling
In the past, data annotation typically involved manual processes, with human annotators going through the data and labeling it according to predefined categories or criteria. This method was particularly common for text annotation tasks, such as highlighting specific entities, marking sentiment, or categorizing text based on topics.
But it was not an ideal method. Manual, traditional data labeling has multiple disadvantages:
Challenges and limitations of manual data labeling
1. They’re time consuming
One of the primary drawbacks of manual data annotation is that it can be incredibly time-consuming. Annotators must go through large volumes of data, which can slow down the development of AI and machine learning models, especially when dealing with complex labeling tasks.
2. They are expensive
Another challenge with traditional data annotation methods is the cost. Hiring and training annotators can be expensive, and the longer the process takes, the more the costs add up, making it less feasible for smaller teams or organizations with limited budgets.
3. They are prone to human error
Manual data annotation is also susceptible to human error, as annotators may make mistakes or interpret data differently. These inconsistencies can negatively impact the quality of the training data, leading to less accurate AI and machine learning models.
4. They are complicated
Traditional data annotation methods often require knowledge of specific programming languages or tools, which can be a barrier for non-technical team members who want to participate in the annotation process. This limitation can exclude valuable insights from domain experts who may not have coding skills but have a deep understanding of the data being annotated.
Introduction to no-code data annotation platforms
What is no-code data annotation?
No-code data annotation platforms are user-friendly solutions designed to streamline the data annotation process without requiring users to have any programming knowledge. These platforms provide intuitive interfaces and tools to label and categorize data, making the annotation process more accessible and efficient for both technical and non-technical users.
Popular no-code data annotation platforms today
How no-code data annotation works
No-code data annotation platforms operate by providing users with a visual interface and pre-built tools to label and categorize data. Users can create custom annotation categories or use predefined ones, depending on their project requirements. Read these tips on how to create good quality text annotation categories.
Once annotation is done, users can then upload their data and start annotating it using the platform's built-in tools, such as entity highlighting, sentiment tagging, or text classification.
Platforms often support collaboration, allowing multiple team members to work on the same dataset simultaneously, ensuring consistent annotations and increasing efficiency.
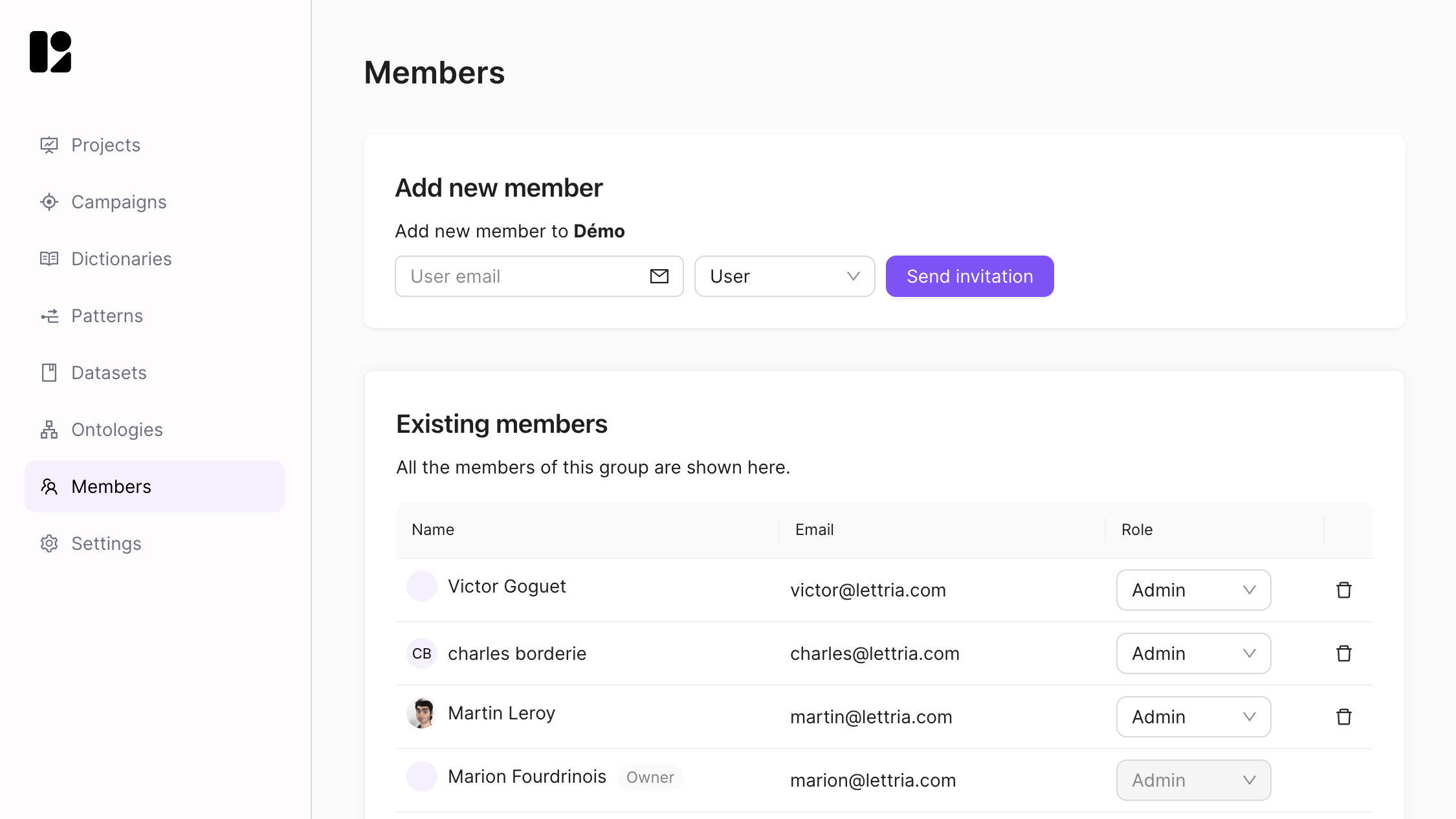
Additionally, no-code data annotation platforms often integrate with existing systems and offer APIs to facilitate seamless data exchange between the platform and the user's machine learning or AI development environment.
Lettria, for example, is an all-in-one platform that includes no-code text annotation as well as model training and deployment.
In-depth look at text annotation
Importance of text annotation in natural language processing
Text annotation is an essential component of natural language processing (NLP), a subfield of AI focused on enabling computers to understand and interpret human language. By labeling and categorizing text data, text annotation provides the necessary context for machine learning algorithms to learn patterns and relationships within the text. This foundation is crucial for the development of applications such as chatbots, sentiment analysis, language translation, and information extraction.
The different types of text annotation
1. Entity recognition
This type of annotation involves identifying and labeling specific entities within the text, such as people, locations, organizations, dates, or other relevant categories. Entity recognition helps machines understand the context and relationships between entities in a text. Learn more about entity recognition in this Lettria article on identifying named entities in a document.
2. Sentiment analysis
Sentiment analysis involves labeling the emotional tone or sentiment expressed in a text, such as positive, negative, or neutral. This type of annotation is useful for understanding the opinions and feelings of users in areas such as product reviews, social media posts, and customer feedback. For more information on sentiment analysis, check out this Lettria article explaining how sentiment analysis works.
3. Text classification
Text classification involves categorizing text into predefined groups or topics. For example, an email could be classified as spam or not spam, or a news article could be labeled as sports, politics, or entertainment. Text classification helps machines organize and filter large volumes of text data.
Challenges in text annotation
1. Ambiguity and context sensitivity
Natural language is often ambiguous, and the meaning of words or phrases can change depending on the context. Annotators must be able to understand the context and accurately label the text, which can be challenging, especially when dealing with idiomatic expressions, sarcasm, or complex sentence structures.
2. Language variations and complexity
Different languages have unique structures, grammar rules, and vocabulary, making text annotation more challenging when dealing with multilingual data. Additionally, regional dialects, slang, and informal language can further complicate the annotation process, as annotators need to be familiar with these variations to accurately label the text. Read more about linguistic variation in NLP on this page.
Advantages of no-code data annotation platforms for text annotation
Time and cost efficiency
Faster annotation process
No-code data annotation platforms, like Lettria, enable faster text annotation compared to manual methods. With intuitive interfaces and built-in tools, users can quickly label and categorize text data, streamlining the development of AI and machine learning models.
Reduced labor costs
By simplifying the annotation process, no-code platforms can reduce labor costs associated with hiring and training annotators. They also minimize the time spent on annotation, which can translate into further cost savings for organizations.
Improved data quality and consistency
Minimizing human error
No-code data annotation platforms help minimize human error by providing standardized tools and guidelines for annotating text. This consistency ensures higher-quality training data, leading to more accurate AI and machine learning models.
Standardized annotation process
These platforms establish a standardized annotation process that helps maintain consistent labeling across different team members and projects. This uniformity is particularly important when working with large datasets or collaborating with multiple annotators.
Scalability and adaptability
Easy integration with existing systems
No-code data annotation platforms often offer APIs or other integration options, allowing them to seamlessly connect with existing systems and workflows. This compatibility makes it easy to scale the annotation process without disrupting existing processes.
Tools like Lettria also include model training and deployment features in the same platform as annotation tools, allowing for seamless movement between tasks.
Ability to handle large volumes of text data
No-code platforms are designed to handle large volumes of text data efficiently, enabling users to annotate and process more data in less time. This scalability is crucial for organizations working with substantial datasets or those aiming to expand their AI and machine learning initiatives.
Accessibility for non-technical users
User-friendly interface
No-code data annotation platforms typically feature user-friendly interfaces that make it easy for non-technical users to annotate text data. This accessibility enables a wider range of team members, including domain experts, to participate in the annotation process.
No programming skills required
As the name suggests, no-code platforms do not require users to have any programming knowledge. This feature allows individuals without coding experience to contribute to the text annotation process, fostering collaboration and leveraging the expertise of diverse team members.
The rise of no-code platforms extends beyond data annotation, with various no-code SaaS solutions addressing IT problems and making technology more accessible to a broader audience.
Lettria: A no-code text annotation solution
Lettria is one such no-code text annotation solution that allows you to annotate your datasets much faster and more efficiently than with manual or traditional solutions.
Features and benefits of Lettria
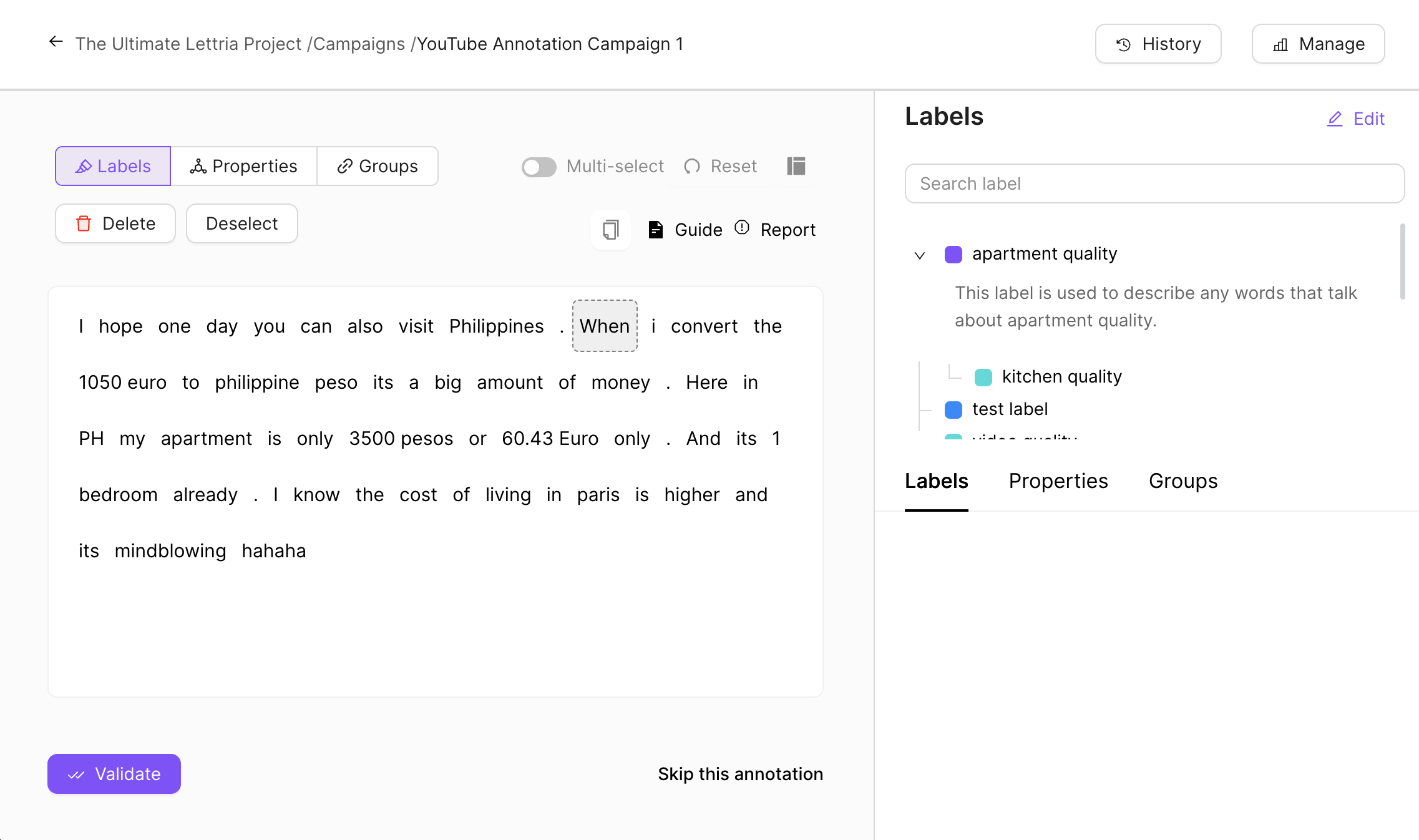
Intuitive interface
Lettria has an intuitive interface that allows anyone, regardless of technical ability, to participate in annotation campaigns. This means that non-technical teams can contribute to the building of an NLP pipeline just as well as technical teams can, resulting in a more robust solution at the end.
Customizable annotation labels
Lettria allows you to easily create a hierarchy of annotation labels that you can use in your annotation campaigns. With an easy to use user-interface, it’s simple to add, edit and delete labels, and organise their hierarchy.
Collaboration and team management
Lettria enables teams to work together on annotation and labeling with its collaboration features. Multiple annotators can work together on the same dataset, and annotations will be validated only when there’s an agreement among a fixed number of annotators.
Use cases and applications of no-code data annotation platforms
Text annotation for natural language processing
No-code data annotation platforms play a vital role in the development of natural language processing (NLP) applications. They facilitate the annotation of text data for tasks such as sentiment analysis, entity recognition, and text classification.
These annotations enable AI and machine learning algorithms to understand and interpret human language, powering applications like chatbots, language translation, and information extraction.
Image annotation for computer vision
Image annotation is another critical use case for no-code data annotation platforms. By labeling objects and features within images, these platforms support the development of computer vision models that can recognize, identify, and track objects.
Applications of image annotation include autonomous vehicles, facial recognition systems, and medical image analysis.
Video annotation for object recognition and tracking
Video annotation involves labeling objects and events in video footage, allowing AI and machine learning algorithms to recognize and track objects over time.
No-code data annotation platforms streamline the video annotation process, making it easier to develop applications like video surveillance, sports analysis, and traffic monitoring.
Custom use cases and industry-specific applications
No-code data annotation platforms are highly adaptable, allowing users to create custom annotation categories and labels that cater to specific industry needs or unique use cases.
This flexibility enables organizations to develop tailored AI and machine learning solutions that address their specific challenges, such as fraud detection in finance, content recommendation in e-commerce, or product defect identification in manufacturing.
Challenges and future developments
Data privacy and security concerns
As no-code data annotation platforms handle sensitive and potentially confidential information, data privacy and security become critical concerns. Organizations must ensure that these platforms comply with data protection regulations and maintain robust security measures to prevent unauthorized access or data breaches.
At Lettria, data privacy and security is a top priority. We continuously work together with all of our clients to ensure that we meet their privacy standards.
Potential limitations
While no-code platforms have numerous advantages, they might not be suitable for every use case or project. Highly specialized or complex tasks may still require custom coding or the expertise of data scientists.
However, ongoing development and advancements in no-code technologies continue to bridge this gap.
Continuous improvement and integration of new technologies
As AI and machine learning technologies evolve, no-code data annotation platforms must also adapt to stay relevant and effective. This includes integrating new techniques and algorithms, improving user interfaces, and expanding the range of supported data types and annotation tasks.
At Lettria, we’re working on integrating LLMs into our text labelling platform in order to make the annotation process even faster, using the capabilities of LLMs to enable zero-shot or few-shot classification.
Conclusion
Recap of advantages
No-code data annotation platforms offer numerous benefits, such as time and cost efficiency, improved data quality, scalability, and accessibility for non-technical users. These advantages are especially valuable in the context of text annotation, a crucial aspect of natural language processing and AI development.
Role of platforms like Lettria
No-code platforms like Lettria are poised to play a significant role in the future of data annotation and AI development.
By making it easier for teams to create high-quality training data, these platforms accelerate the development of AI solutions and enable organizations to harness the power of AI and machine learning more effectively.
Sign up for Lettria to explore no-code text annotation and model training
If you're looking to streamline your data annotation process, especially in the realm of text annotation, consider exploring no-code data annotation platforms like Lettria. By harnessing the power of no-code solutions, you can accelerate your AI development process and build more accurate, efficient models to drive your organization's success.