9 min
Introduction
To enable companies to obtain relevant information from large volumes of unstructured data, Lettria has developed its GrapRAG solution, actually a hybrid RAG on both vector and graph stores. The innovative combination of these two methods delivers impressive results, far superior to those obtained with the usual vector-based approach alone. To prove this value, we have launched a series of benchmarks with Q&A on complex documents and an orderly process. The data in question has been selected to form several corpora representative of different business sectors. The questions asked are based on a very comprehensive framework, and the evaluation of responses is based on a precise grid.
Process
The process is simple. With Lettria’s platform, we ingest the same data through two different pipelines:
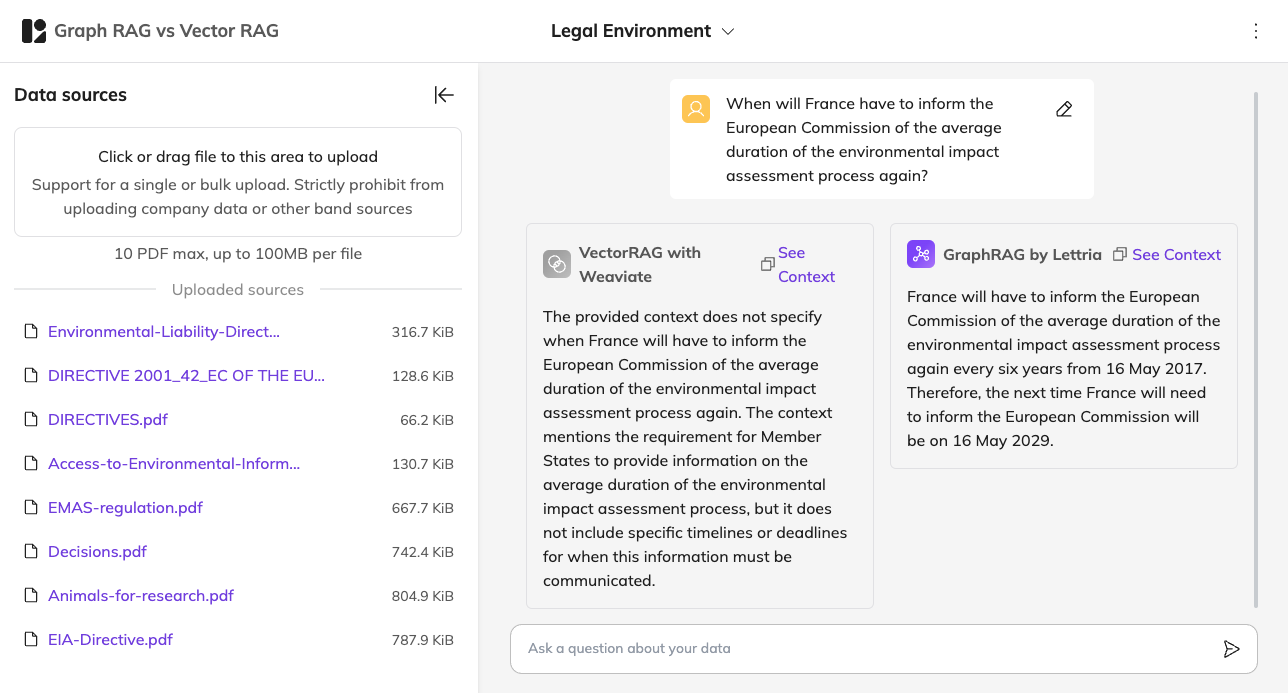
Then we test the prepared questions and analyze the answers. Lettria's platform not only enables immediate comparison of results, but also deeper analysis thanks to the “See context” buttons, which give access to the chunks used by both methods to provide the answer, as well as to the generated graphs in the case of GraphRAG.

Representative use cases
Four benchmarks were conducted with written corpora specific to different fields of activity and which correspond to use cases representative of the needs of companies or public bodies. The sectors selected are:
- Finance, with reports from Amazon.
- Health, with scientific texts on vaccines against covid-19.
- Industry, with technical specifications concerning construction materials in aeronautics.
- Law, with European directives concerning the environment.
Question typology
For each benchmark, the questions asked respond to a precise typology. Here are the six types.
Factoid questions
These are trivia-style questions with a single, clearly defined, and factually correct answer. For example, for the health sector, we wrote the question “What is the formula of the MigVax-101 vaccine?”. The answer can be found in a single extract from a single document: “MigVax-101 is a multi-antigen vaccine that contains the RBD and two domains of the N from SARS-CoV-2 and heat-labile enterotoxin B (LTB) as a potent mucosal adjuvant”.
Multi-hop questions
These questions demand a comprehensive understanding that involves combining insights from various sections that may come from a single document or from several documents. For example, still in the healthcare field, the following question was asked: “Is there any difference between Moderna and BioNTech RNA vaccines ?”. To give the right answer, you need to combine information from different parts of two documents concerning different aspects of these two vaccines: their stability, their storage duration, their efficacy, their side effects, etc.
Numerical reasoning questions
These are questions involving counting, comparisons, or calculations. If we ask a question such as “How would the elastic modulus and density change if 1.5% Li is added to an Al-Li alloy?”, you need to make calculations based on the information provided in the documents. In this case, it is the percentage increase in elastic modulus and the percentage increase in density for every 1% of Li added, which must each be multiplied by 1.5 to obtain the correct answer.
Tabular reasoning questions
These questions involve information or statistics found in tables. To answer the question “What is the minimum surface area required for a 7500 gram turkey?”, you'll need to look up the information in the following table and find the number in the right column on the right line.

Temporal reasoning questions
These are questions involving reasoning through timelines. Take, for example, the question “When will France have to inform the European Commission of the average duration of the environmental impact assessment process again?”. To find the right answer, first look for the following information: “In particular, every six years from 16 May 2017 Member States shall inform the Commission, where such data are available, of (...) the average duration of the environmental impact assessment process”. We then need to calculate the following deadlines and take into account the fact that the question is asked in 2024 in order to provide the correct answer: 2029.
Multiple constraints questions
These questions involve several of the constraints listed above: temporal, tabular, numerical, etc. Such is the case with this query: “Compare the oldest booked Amazon revenue to the most recent”. We need to find the amounts in question, located in different documents, which corresponds to a multi-hop question, and calculate the progression between the two, i.e. answer a numerical reasoning question.
Evaluation grid
To best analyze the results and compare the two solutions, we used a very precise grid. It is made up of the following categories and subcategories:
Correct answers:
- Correct.
- Incomplete answer because the sources have not been uploaded in the collection.
- Correct but answer too long or with too many details.
Partially correct but acceptable answers:
- Correct but repetitions in the answer.
- Correct but quite incomplete (the source was uploaded in the collection).
- Correct but including irrelevant information that is not misleading.
Partially correct but not acceptable answers:
- The sources used are wrong or too incomplete.
- The question is not understood.
Incorrect answers:
- The sources used are wrong and lead to a wrong answer.
- The sources were not found.
- Hallucination.
Results
General results
The overall analysis of results is edifying. 80% of queries are answered correctly with GraphRAG, compared with 50.83% with VectorRAG. These figures rise to almost 90% respectively, versus 67.5% if we add up acceptable responses to correct responses.

Results by sector
If we analyze the results of each benchmark independently, we can see that GraphRAG's results are better in all areas, with a significant gap with VectorRAG's results in all cases, almost doubling the number of correct answers in the case of industry: 90.63% versus 46.88%. It is in this field that GraphRAG offers the most correct answers, and in finance if we add the acceptable answers: 96.42%.
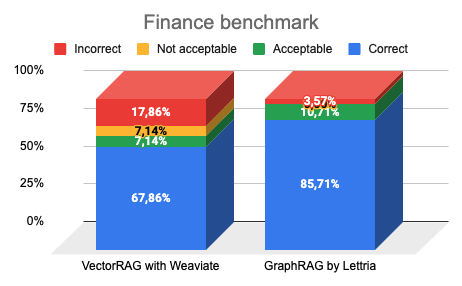



Results by question type
If we now classify the results by question type, we can see that GraphRAG is still better, except for tabular reasoning questions, where its results are identical to those of Vector RAG: 33% correct answers and 33% acceptable answers in both cases. The Lettria teams are actively working on a better analysis of this type of data, and GraphRAG's results will certainly be better in future evaluations.
For other question types, GraphRAG's results are clearly superior. It achieves 92% correct answers for numerical reasoning questions, and the biggest discrepancy concerns temporal reasoning questions: 83.33% correct answers for GraphRAG, while VectorRAG has neither correct nor acceptable answers. GraphRAG is clearly superior in complex cases, when it comes to cross-referencing information and reasoning.


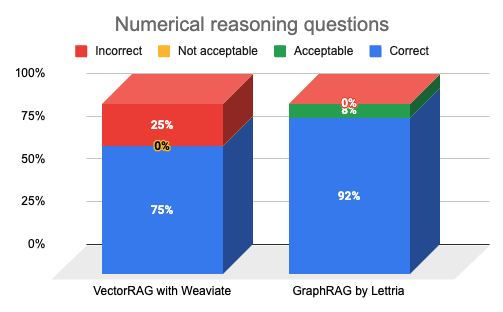
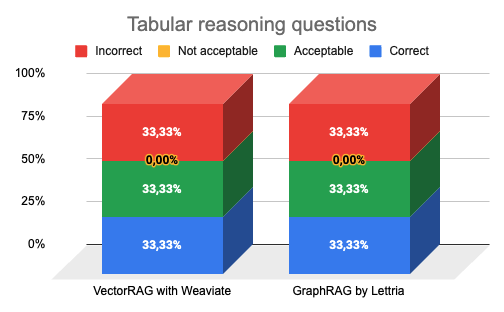


Conclusion
The benchmarking results highlight how Lettria's GraphRAG offers substantial advantages over the VectorRAG approach, particularly for clients focused on structuring complex data. GraphRAG’s overall reliability and superior handling of intricate queries allow customers to make more informed decisions with confidence, ultimately boosting their efficiency and reducing the time spent sifting through unstructured data.